About
The connection between causality and AI/ML has become increasingly important in recent years, as more and more organizations seek to use AI/ML to make decisions in a variety of domains that like healthcare, finance, transportation, and more. While recent data-driven AI techniques, especially deep representation learning, have shown great promise in areas that involve data analysis, they have also been criticized for their lack of interpretability, explainability, and transparency, which can limit the trustworthiness, accountability, and reliability of AI/ML systems. Additionally, the correlations embedded in the data, which is identified by current cutting-edge models such as GPT-4 and Llama do not necessarily imply causation, which can further lead to issues like spurious correlations or algorithmic bias when such mismatch are not properly explained and addressed. Recently, causal representations have shown great potential in understanding the generating process behind data and provide a framework for understanding how machine learning models make decisions through investigating the underlying causal relationships between variables. By incorporating causal representation learning (CRL), researchers and practitioners can better understand how different features or inputs contribute to the outcome of interest and identify potential sources of bias or confounding that could affect the decision-making and further generalizations.
Moreover, from a data-centric perspective, this workshop will focus on both automatic knowledge construction utilizing causal representations discovered from pure data and knowledge enhancement based on domain expert knowledge in real-world applications. Leveraging these causal insights, practitioners can strengthen the robustness and reliability of AI/ML systems deployed in real problems, ensuring that decisions made by the intelligent system are not only data-driven but also grounded to a deeper understanding of the causal mechanism behind.
In this workshop, we discuss both theoretical and applied aspects of CRL, which includes but not limited to the following topics:
-
Theory of causal representation learning
-
Causal representation learning models
-
Causal generative models
-
Causal foundation models
-
Causal discovery with latent variables
-
Causal representation learning for treatment effect estimation
-
Causal representation learning in data mining
-
Causal representation learning in recommendation systems
Keynote Speakers
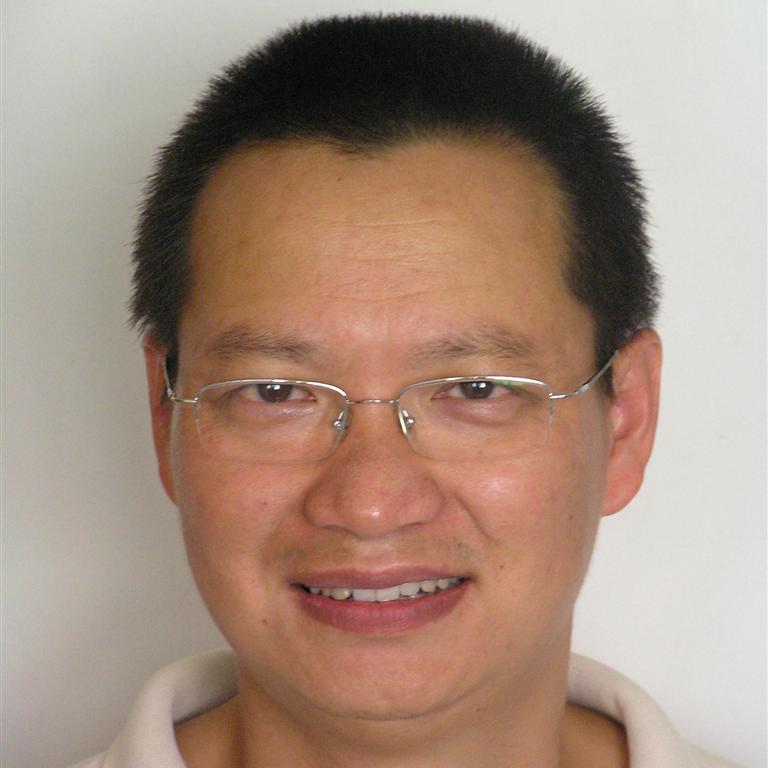
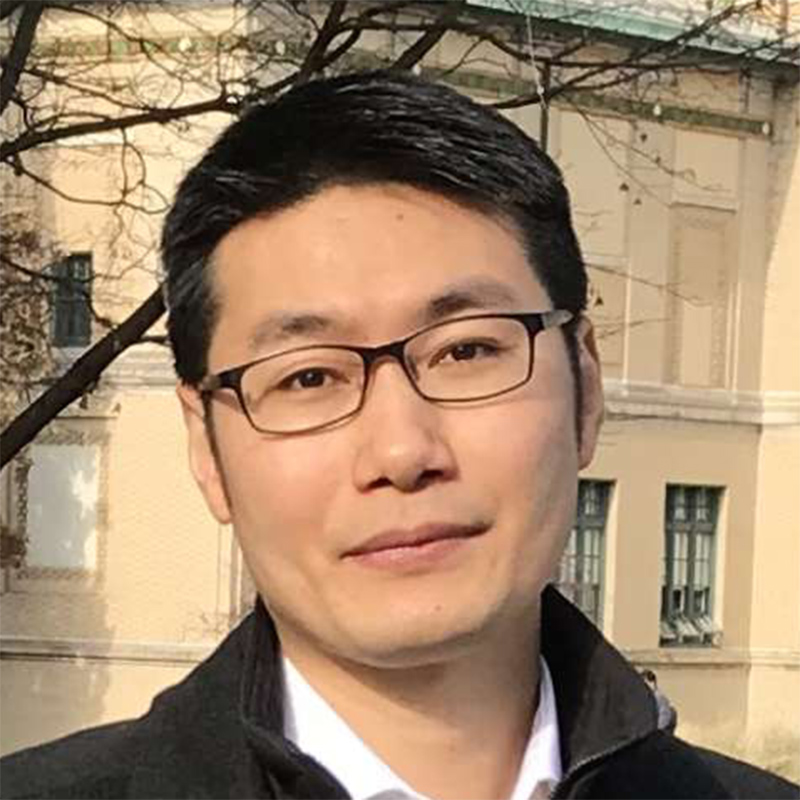
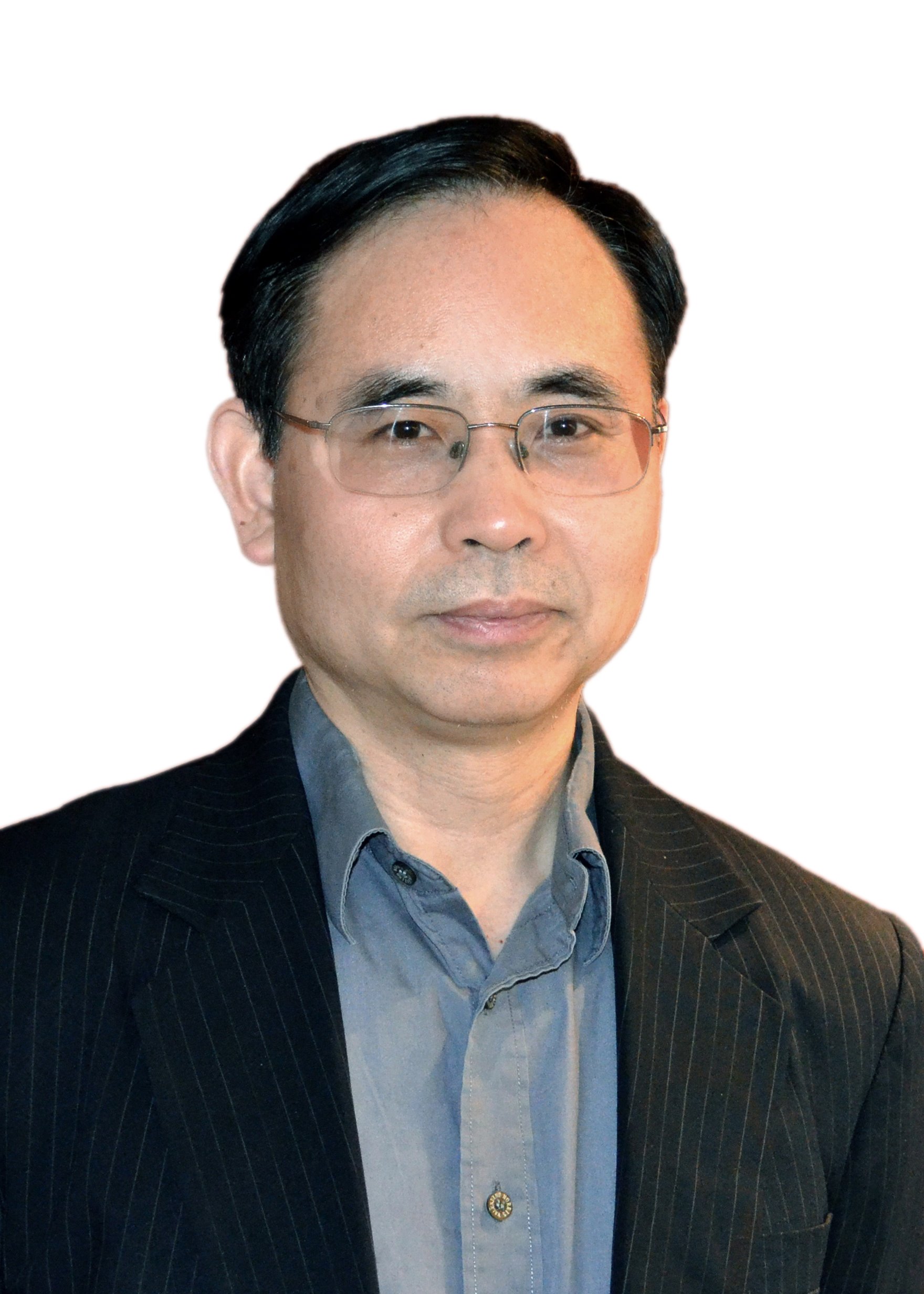
Tentative Schedule
- 8:00-8:05 AM: Welcome Remarks
- 8:05-8:35 AM: Keynote Talk 1 by Prof. Xiao-Hua Zhou, Peking University
- 8:35-9:05 AM: Keynote Talk 2 by Prof. Jiuyong Li, University of South Australia
- 9:05-9:15 AM: Contributed Talk 1 by Tianjun Yao, MBZUAI (Title: Learning Invariant Features on Graphs by Disentangling Spurious Features)
- 9:15-9:25 AM: Contributed Talk 2 by Chunyuan Zheng, Peking University (Title: UMVUE-DR: Uniformly Minimum Variance Unbiased Doubly Robust Learning with Reduced Bias and Variance for Debiased Recommendation)
- 9:25-9:55 AM: Keynote Talk 3 by Prof. Kun Zhang, CMU and MBZUAI
- 9:55-10:05 AM: Contributed Talk 3 by Han Qiao, South China Normal University (Title: Deep Entire Space Cross Networks for Estimating Treatment Effect Under Unmeasured Confounding)
- 10:05-10:30 AM: Coffee Break
- 10:30-11:00 AM: Keynote Talk 4 by Prof. Amin Beheshti, Macquarie University
- 11:00-11:10 AM: Contributed Talk 4 by Xinghang Hu, Sichuan University (Title: M3-InvRL: Multimedia Model Merging Invariant Representation Learning for Recommendation)
- 11:10-11:20 AM: Contributed Talk 5 by Haotian Wang, National University of Defense Technology (Title: Learning Feasible Causal Algorithmic Recourse without Prior Structure)
- 11:20-11:30 AM: Contributed Talk 6 by Meilin Wang, Renmin University of China (Title: Projection Pursuit Based Density Ratio Estimation)
- 11:30-12:00 PM: Panel discussion
- 12:00-12:10 PM: Closing Remarks
Important Dates (Anywhere on Earth)
- Workshop Papers Submission:
September 10, 2024October 20, 2024 - Acceptance Notification: November 7, 2024
- Camera-ready Deadline and Copyright Form: November 11, 2024
- Workshop Date: December 9, 2024
Submissions
Please submit your manuscripts on Open Reivew.
Organizers:
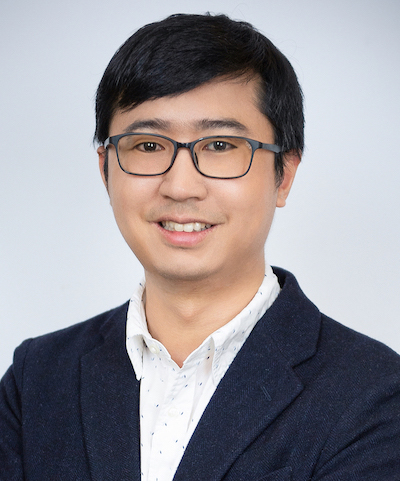
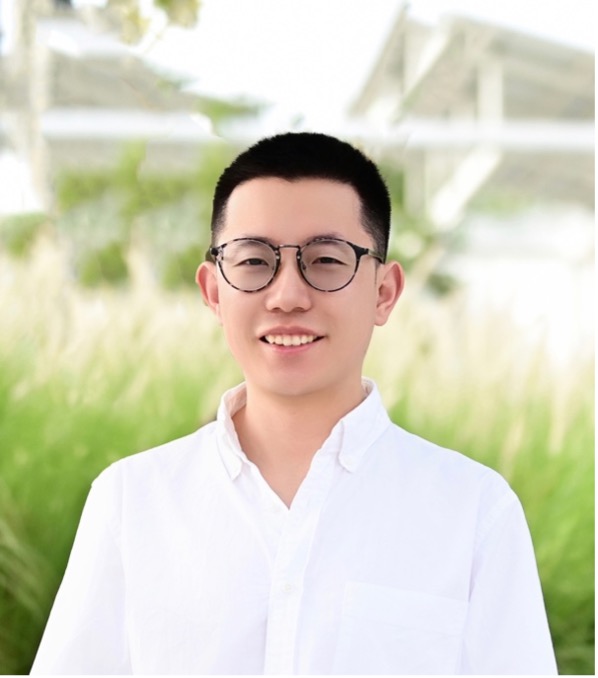
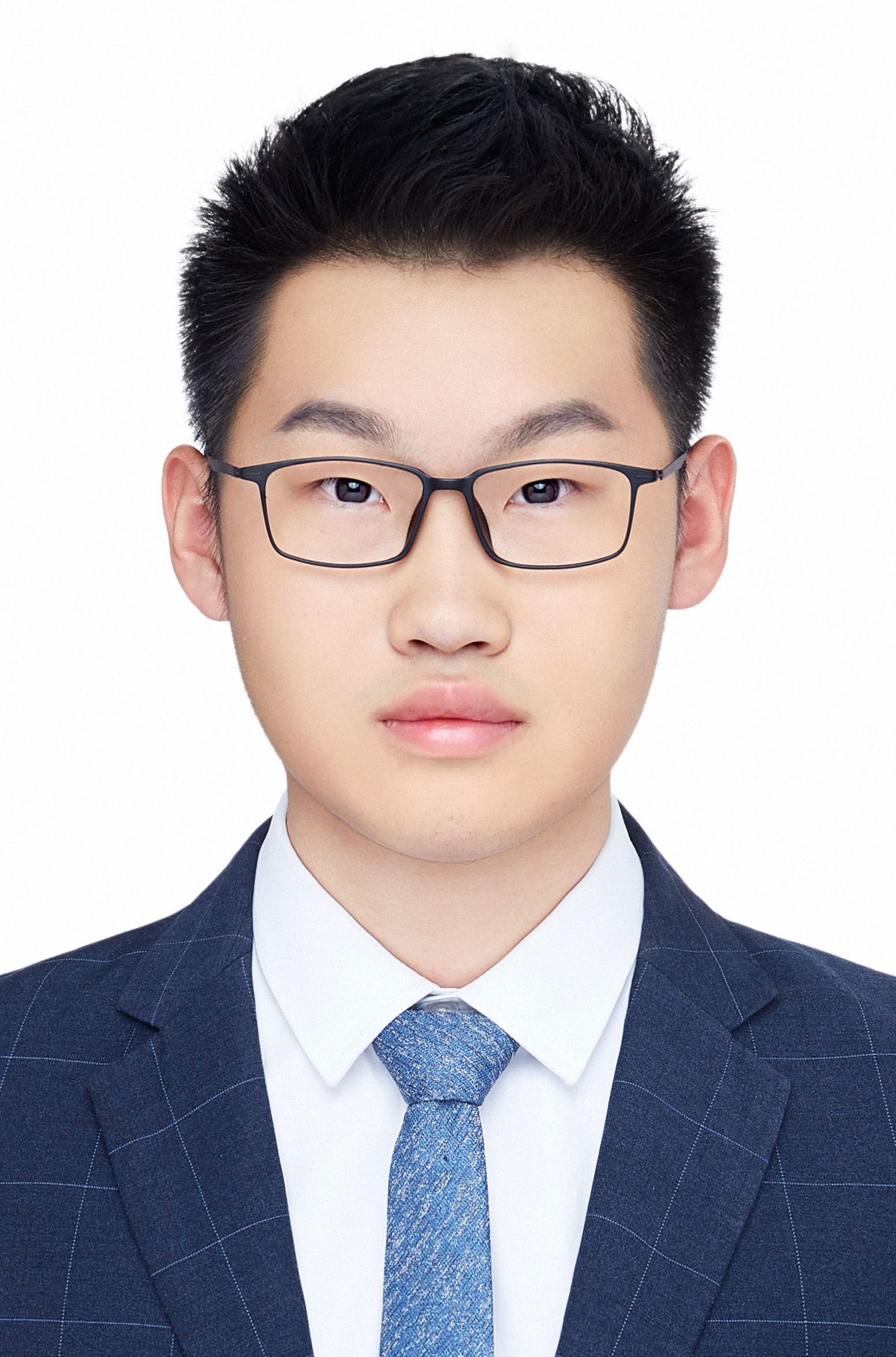
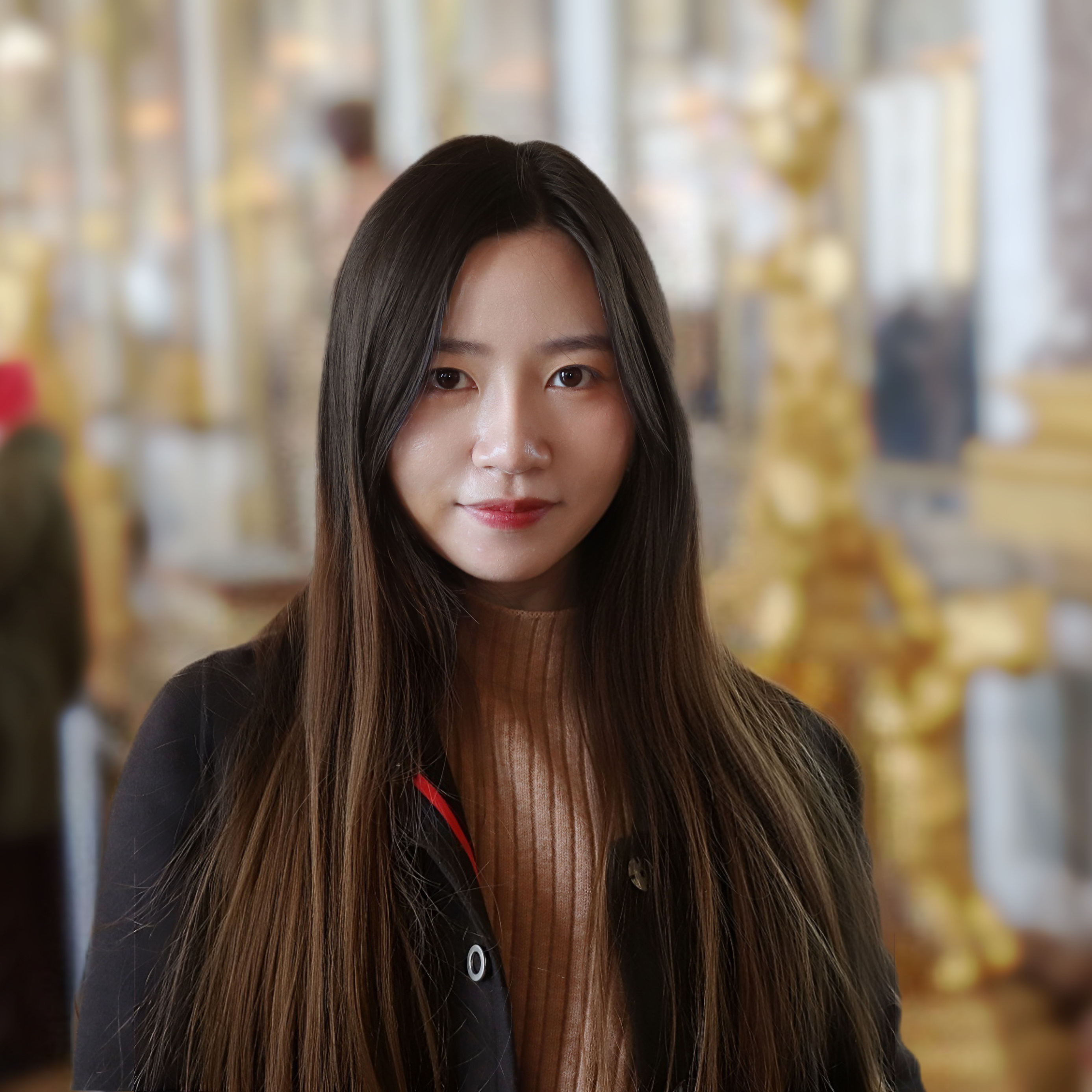
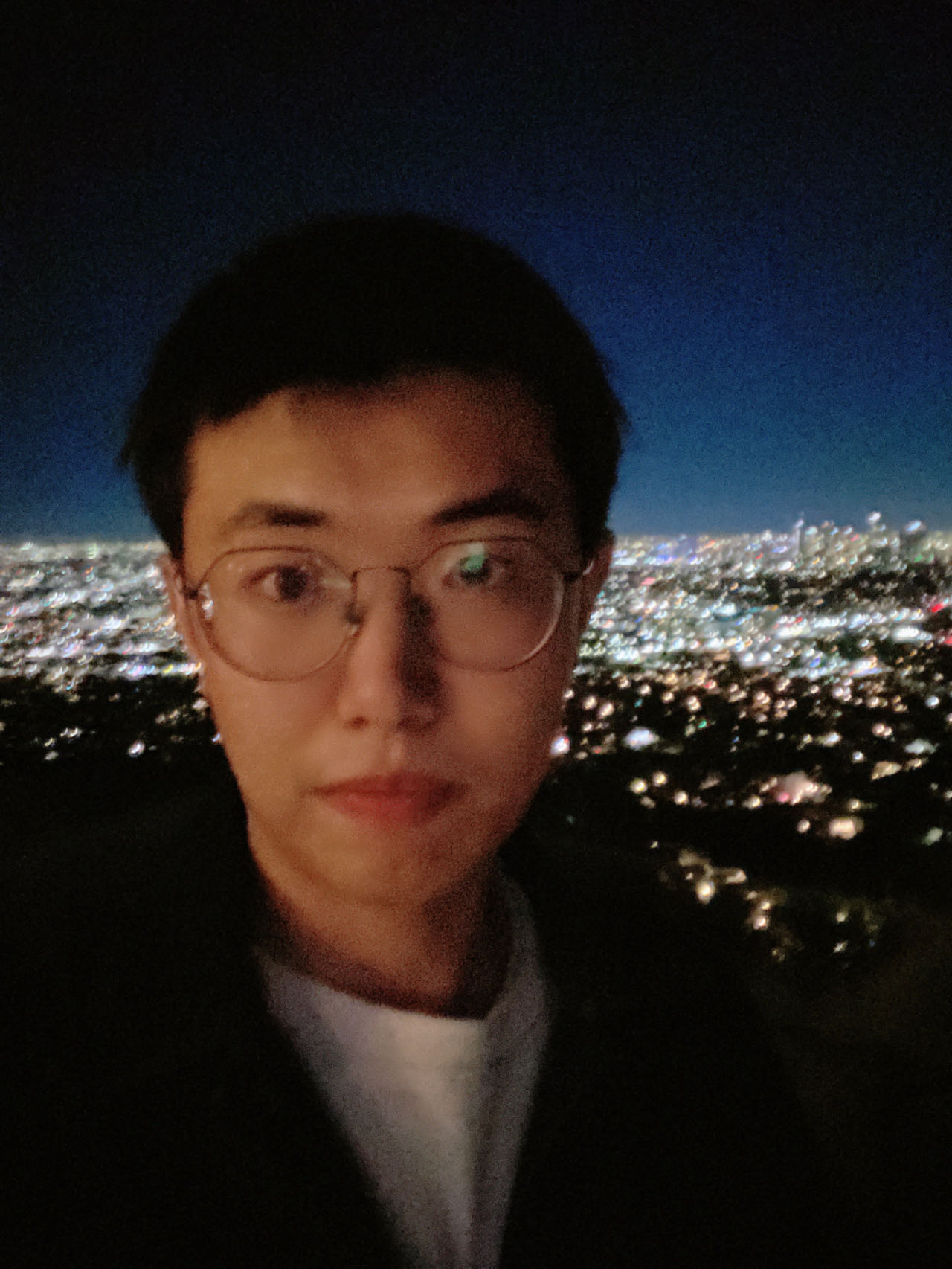
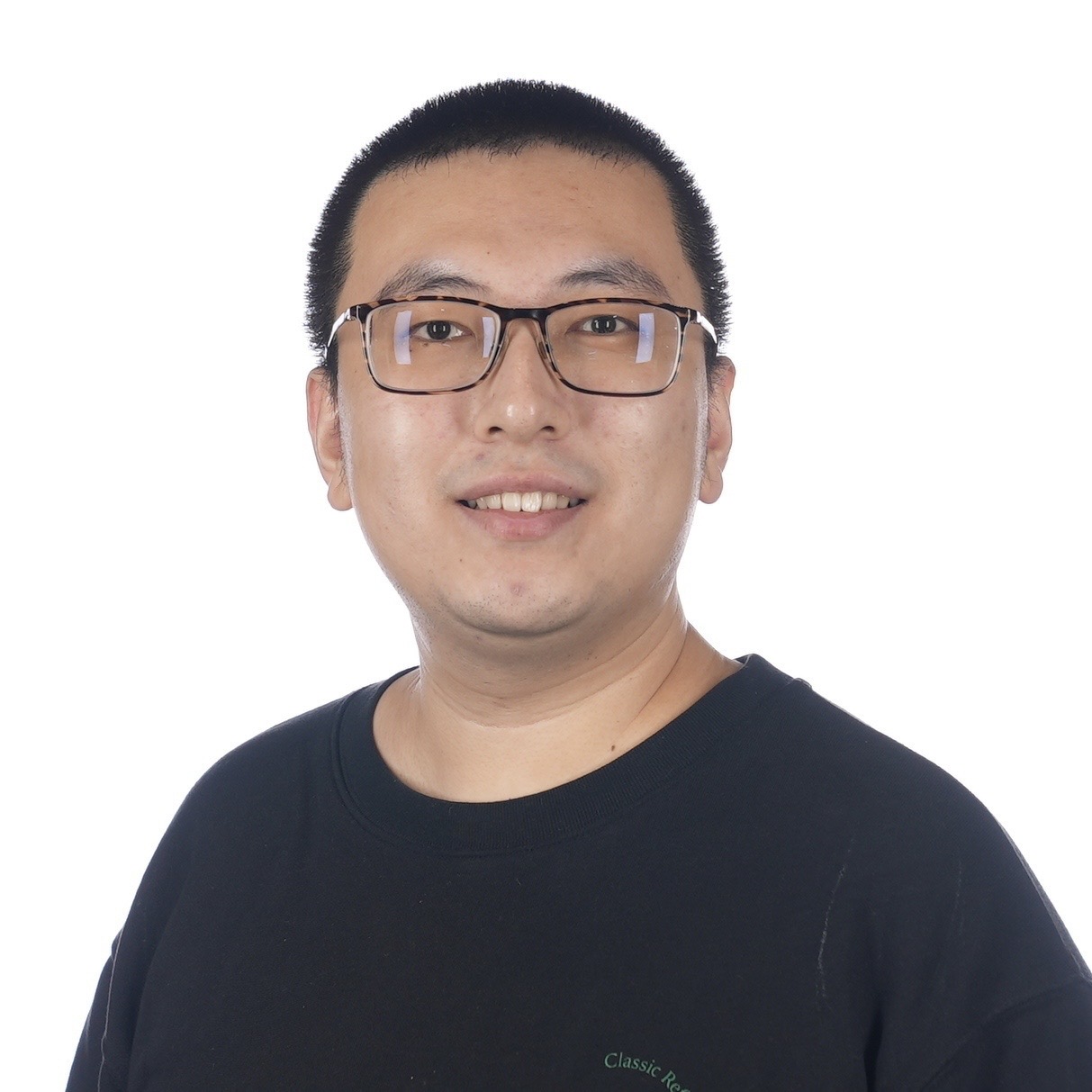
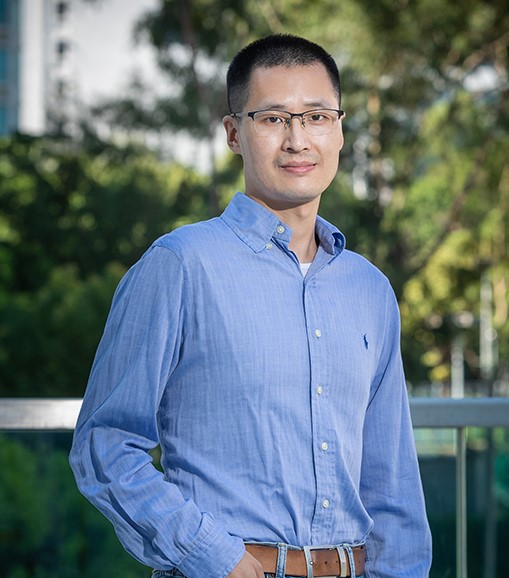
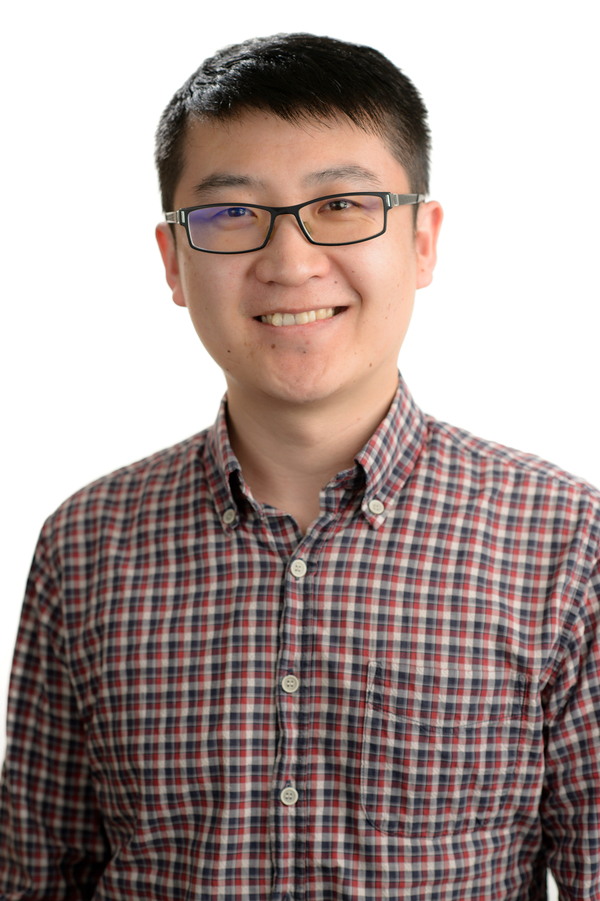